SEACells: an algorithm for identifying metacells
Scientists have identified a disconnect between the cellular resolution of single-cell genomics data and the cluster-level resolution of analysis, which has limited the utilization of this data in biomedical research. Typically, a dataset that contains enormous information on tens of thousands of cells is compressed by clustering to overcome the noise and sparsity characteristics of single-cell data.
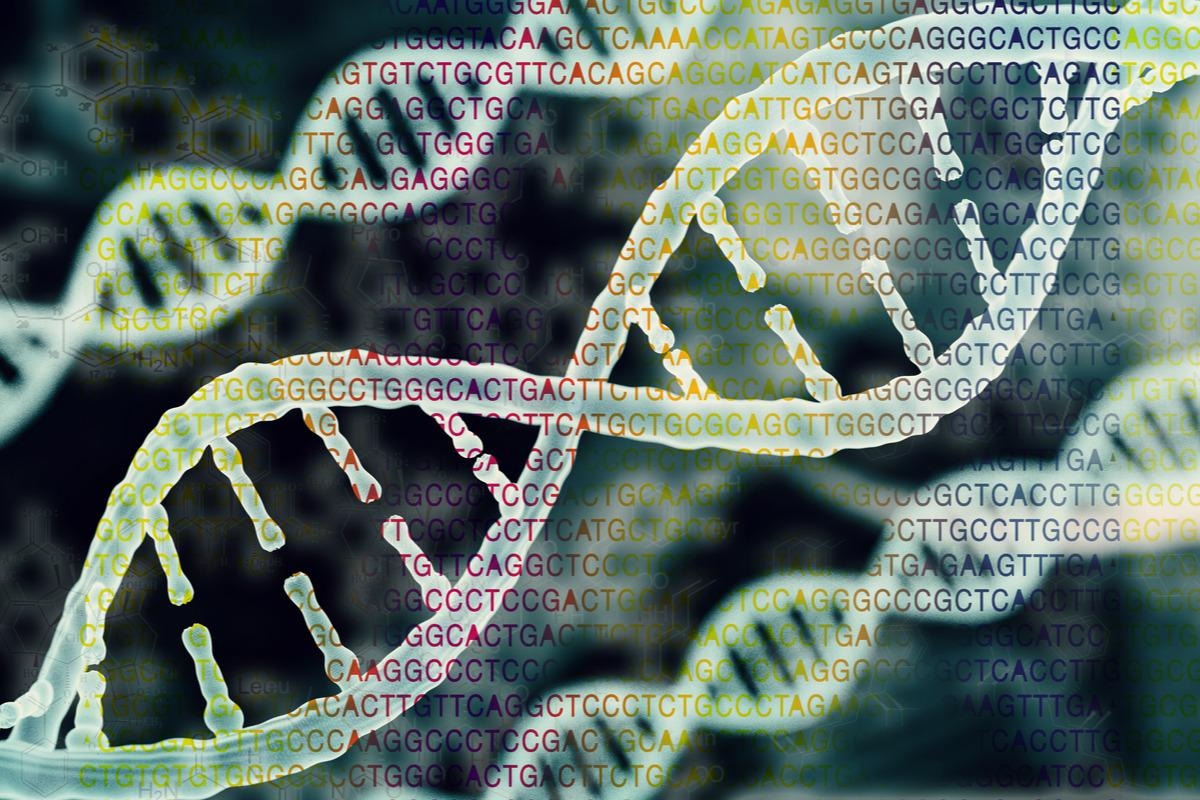
Background
Acute sparsity has been associated with a single-cell assay for transposase-accessible chromatin sequencing (scATAC-seq) data. This data captures the trinary zygosity states at a few thousand of the hundreds of thousands of open chromatin regions in a cell, making it extremely difficult to determine regulation at the single-cell level.
Although single-cell RNA sequencing (scRNA-seq) data is not as sparse, studies on the Human Cell Atlas and Human Tumor Atlas Network contain millions of cells. A large number of cells pose difficulty in routine analysis related to dimensionality reduction and visualization. This is the reason why large scRNA-seq are analyzed at a cluster level.
Scientists revealed that cluster-level analysis has resulted in many important biological discoveries. Typically, a cluster is not homogenous, but it possesses a structured variability in gene programs. For instance, cells within T-cell clusters display variable activation and metabolic functions.
Metacells are groups of cells representing singular cell states from single-cell data. The concept of metacells has been associated with diverse and highly granular cell states. The variation within metacells occurs due to technical variability and not biological factors. Researchers have stated that metacells are more granular than clusters, and are optimized for homogeneity within cell groups. The available approaches have not been successful for scATAC-seq data and are poorly distributed across the phenotypic space. Scientists have also pointed out that metacells are immensely underutilized in single-cell analysis, especially since scATAC-seq data has remained unexplored.
A new study
A new study published on bioRxiv* preprint server has presented single-cell aggregation of cell-states (SEACells), a graph-based algorithm for identifying metacells. SEACells utilizes iterative archetypal analysis to compute metacells. The authors of this study tested their algorithm on peripheral blood data (distinct and well-separated cell types). In addition, the effectiveness of SEACells was also evaluated using CD34+ hematopoietic stem and progenitor cell (HSPC) data from human bone marrow.
One of the assumptions of the SEACells algorithm is that all biological systems consist of well-defined and finite sets of cell states. The observed single-cell data contains a high degree of noise, and the cells samples from the same states are assumed to be closely linked to their phenotypes owing to their similar gene expression patterns and regulatory mechanisms. SEACells algorithm focuses on aggregating single cells that are closely linked and identifying metacells that represent cell states. Owing to aggregation, metacells overcome the issues related to sparsity as well as retained heterogeneity.
Some of the key inputs of the SEACells algorithm are raw count matrices, which involve gene expression for RNA, etc., low dimensional representation of the data, and the number of metacells to be identified. SEACells utilize these inputs to generate output groupings of cells that represent metacells.
Key findings
The authors revealed that SEACells metacells provided comprehensive characterizations of scRNA-seq cell states, which included information about gene-gene relationships representative of each state. It can also characterize scATAC-seq datasets and in principle, can be applied to other single-cell modalities. Furthermore, this algorithm can describe chromatin cell states which are useful for deciphering regulatory elements associated with underlying gene expression.
Importantly, scientists revealed that these metacells not only offered a sweet spot between signal aggregation and cellular resolution, but they also captured cell states across the phenotypic spectrum, including rare states.
One of the main advantages of the design principle on which the SEACells algorithm is based is that it ensures the identification of metacells that are compact, well separated, and span the entire phenotypic manifold. As data obtained are computationally tractable, researchers are able to perform downstream analysis of large-scale datasets.
Researchers have used SEACells to understand the dynamics of expression and accessibility related to hematopoietic differentiation that occurs in COVID-19 infection. They further determined temporal dynamics of T-cell response during the infection. Scientists revealed biological functions that are typically missed by single-cell and cluster-level analysis. Additionally, the authors stated that metacells can be computed separately for each sample, and integration of additional cohorts is possible, which renders heterogeneity in the data.
Conclusion
The authors stated that SEACells provides a robust toolkit to analyze genetic interferences using scATAC-seq data. To date, only this toolkit has been able to derive cell states from scATAC-seq data accurately and comprehensively. It also provides a solution for the integration of large cohort-based single-cell data.
*Important notice
bioRxiv publishes preliminary scientific reports that are not peer-reviewed and, therefore, should not be regarded as conclusive, guide clinical practice/health-related behavior, or treated as established information.
- Persad, S. et al. (2022) "SEACells: Inference of transcriptional and epigenomic cellular states from single-cell genomics data". biRxiv. doi: 10.1101/2022.04.02.486748. https://www.biorxiv.org/content/10.1101/2022.04.02.486748v1
Posted in: Device / Technology News | Medical Science News | Medical Research News
Tags: Assay, Blood, Bone, Bone Marrow, Cell, Chromatin, covid-19, Gene, Gene Expression, Genetic, Genomics, Research, RNA, RNA Sequencing, Single-cell genomics, T-Cell, Tumor
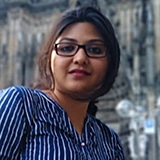
Written by
Dr. Priyom Bose
Priyom holds a Ph.D. in Plant Biology and Biotechnology from the University of Madras, India. She is an active researcher and an experienced science writer. Priyom has also co-authored several original research articles that have been published in reputed peer-reviewed journals. She is also an avid reader and an amateur photographer.
Source: Read Full Article