Machine learning predicts hospital-onset COVID-19 infections using patient contact networks
Accurate and real-time disease prediction is vital for the prevention and control of healthcare-related infections. Although contacts between individuals are primarily responsible for infection chains, most prediction frameworks do not capture the contact dynamics.
A real-time framework using patient contact networks to predict hospital-onset COVID-19 infections
Researchers from the UK recently developed a real-time machine learning framework that uses dynamic patient contact networks to predict hospital-onset COVID-19 infections (HOCIs) at the patient level. They then tested and validated the framework on international multi-site datasets across various epidemic and endemic periods. This study can be found on the medRxiv* preprint server.
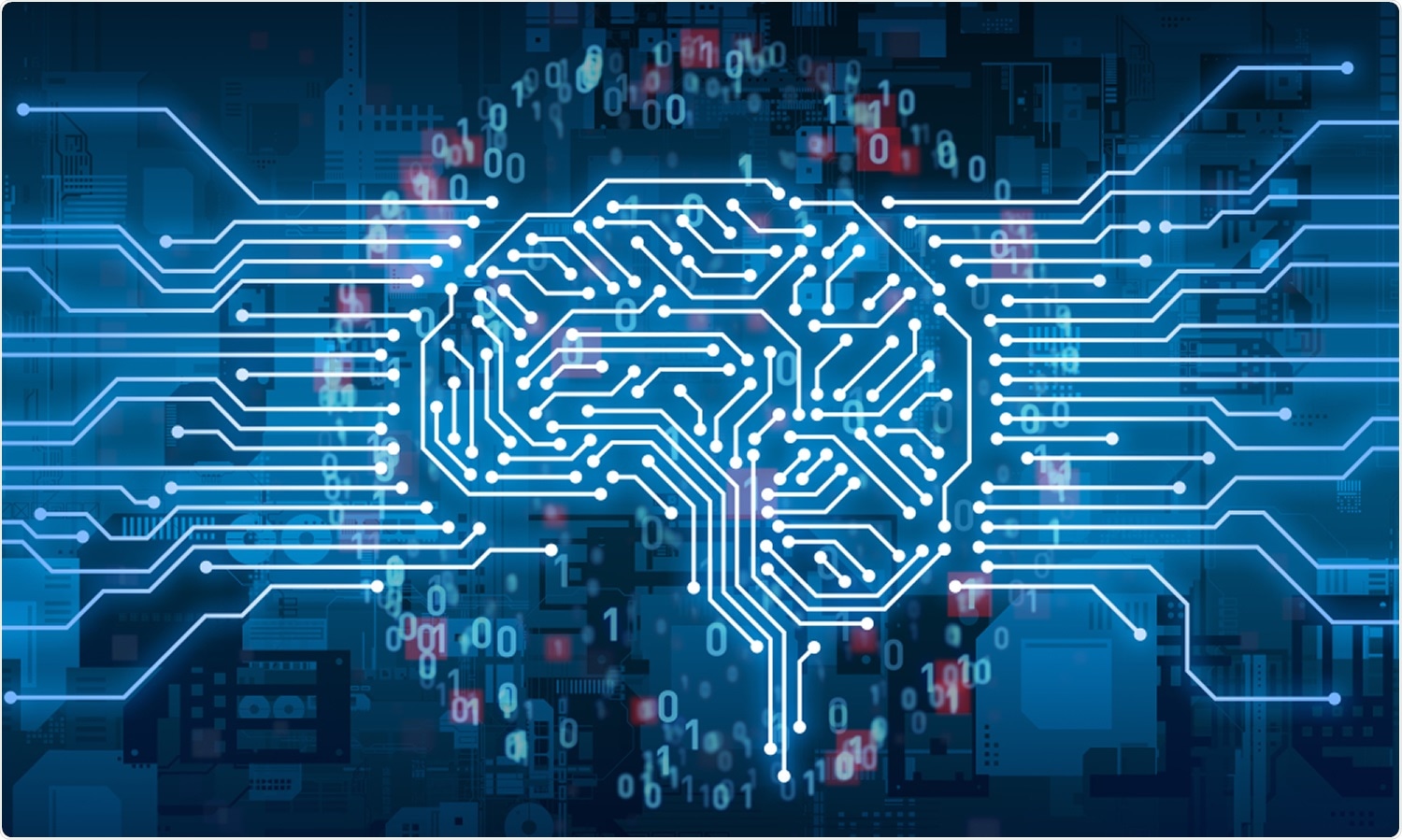
The framework extracts dynamic contact networks from hospital data collected routinely and combines them with clinical attributes and background contextual hospital data of the patient to predict the infection status of patients. The research team trained and tested the HOCI framework using over 50,000 patients admitted to a UK National Health Service (NHS) Trust hospital between 01 April 2020 and 01 April 2021, thus spanning COVID-19 surges 1 and 2 in the UK.
They then validated the HOCI framework by applying it to data from 40,057 inpatients gathered from a hospital site in Geneva during an epidemic surge and data from 43,375 inpatients collected from the same NHS Trust from a subsequent period after surge 2, when COVID-19 had become endemic.
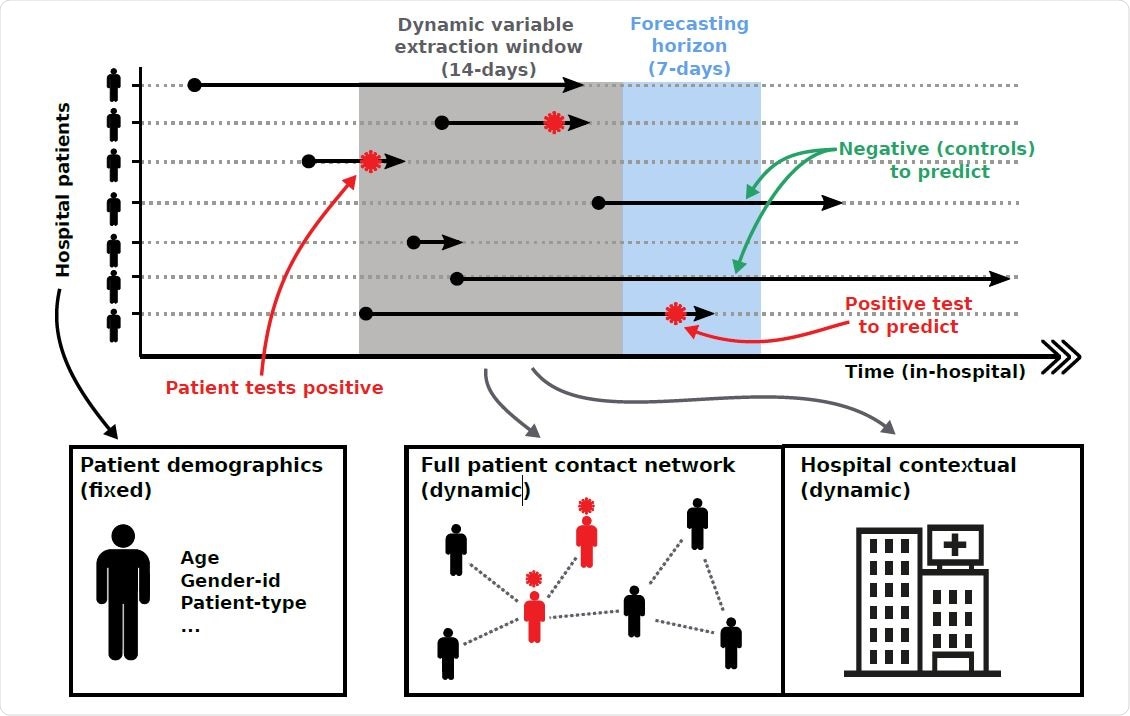
The HOCI framework demonstrated high predictive performance while using only contact network variables
Based on the training data from London spanning COVID-19 surges 1 and 2, the HOCI framework showed high predictive performance using all variables but was almost equally predictive using only contact network variables and more predictable than using only hospital contextual or patient clinical variables.
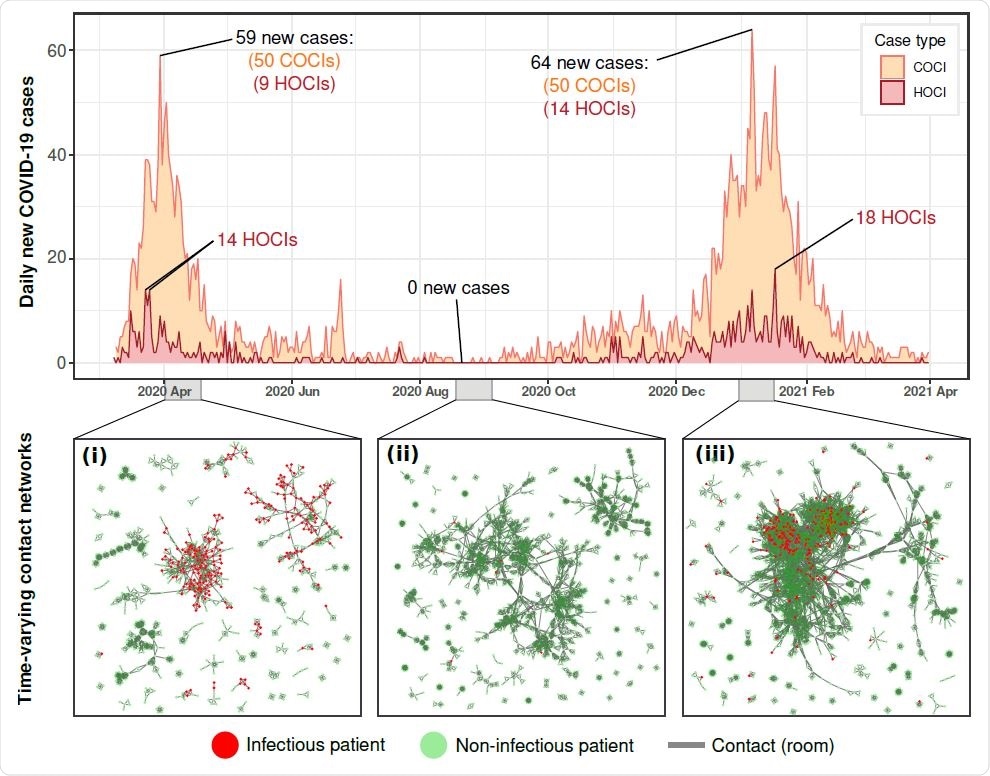
The top 3 risk factors identified by the team comprised one hospital contextual variable, namely, background hospital COVID-19 prevalence, and two contact network variables, namely, the number of direct contacts of infectious patients and network closeness. Moreover, the addition of contact network variables boosted performance related to hospital contextual variables on both the UK and non-UK validation datasets.
“Our study highlights the predictive power that can be mined from networks of patient contacts to aid with personalized predictions of infectious disease in healthcare settings.”
Findings show that integration of patient contact networks in clinical care could improve infection prevention and help early diagnosis
According to the authors, this is the first study to predict individual patient HOCIs using routine patient and hospital data and dynamic contact networks. The research team combined machine learning with network analysis and developed a HOCI prediction framework with the help of routine electronic health data.
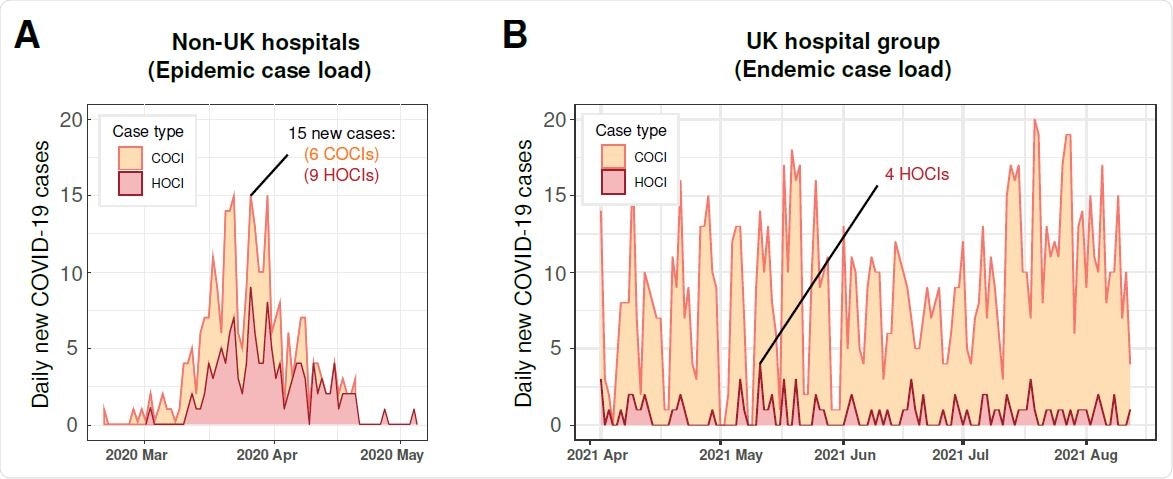
The results showed that dynamic patient contact networks could be a strong predictor of transmission of respiratory viral infections in hospitals. Thus, the integration of these networks in clinical care could improve individualized prevention of severe acute respiratory syndrome coronavirus 2 (SARS-CoV-2) infection as well as early diagnosis.
“The framework provides daily patient-individualized predictions and can identify dynamic disease acquisition risk factors.”
They found that centrality measures calculated from patient contact networks together with some hospital contextual variables represent a key HOCI risk factor, which can boost the performance of predictive models in a pandemic as well as endemic conditions.
To demonstrate the transferability of the framework, they applied it to data from a Geneva-based hospital during COVID-19 surge 1 and showed increased predictive power by including contact network risk factors. However, access was only available for room-level contacts that were less predictive in the London data.
With no end in sight to the COVID-19 pandemic, this new predictive framework and contact risk factors offer a valuable tool to assist identification of potential HOCI cases across various epidemic and endemic periods.
“Further work will be needed to extend this work to other healthcare-acquired infections by assessing how the inclusion of variables capturing a patient’s environment within an underlying contact network could aid prediction with a view to informing infection prevention and control measures.”
*Important Notice
medRxiv publishes preliminary scientific reports that are not peer-reviewed and, therefore, should not be regarded as conclusive, guide clinical practice/health-related behavior, or treated as established information.
- Predicting hospital-onset COVID-19 infections using dynamic networks of patient contacts: an observational study Ashleigh Myall, James R Price, Robert L Peach, Mohamed Abbas, Siddharth Mookerjee, Nina Zhu, Isa Ahmad, Damien Ming, Farzan Ramzan, Daniel Teixeira, Christophe Graf, Andrea Y Weiße, Stephan Harbarth, Alison Holmes, Mauricio Barahona medRxiv 2021.09.28.21264240; doi: https://doi.org/10.1101/2021.09.28.21264240, https://www.medrxiv.org/content/10.1101/2021.09.28.21264240v1
Posted in: Device / Technology News | Medical Science News | Medical Research News | Disease/Infection News | Healthcare News
Tags: Coronavirus, Coronavirus Disease COVID-19, Epidemiology, Healthcare, Hospital, Machine Learning, Pandemic, Research, Respiratory, SARS, SARS-CoV-2, Severe Acute Respiratory, Severe Acute Respiratory Syndrome, Syndrome

Written by
Susha Cheriyedath
Susha has a Bachelor of Science (B.Sc.) degree in Chemistry and Master of Science (M.Sc) degree in Biochemistry from the University of Calicut, India. She always had a keen interest in medical and health science. As part of her masters degree, she specialized in Biochemistry, with an emphasis on Microbiology, Physiology, Biotechnology, and Nutrition. In her spare time, she loves to cook up a storm in the kitchen with her super-messy baking experiments.
Source: Read Full Article